A/B Testing Best Practices | Segment Growth Center
We go over the A/B testing best practices and how to use this method to optimize your website and marketing campaigns.
An A/B test is when you change one element of a webpage, app, or digital asset, to see if this impacts user behavior. The point of an A/B test is to better understand how content, campaigns, or even UX design is influencing customer decision-making and the quality of your user experience.
While all experiments begin with a hypothesis, the most successful experimentation campaigns have clear metrics and KPIs attached (i.e. how are you measuring success?).
We’ve provided a few best practices for A/B testing below, to ensure that your team is leveraging experimentation to drive optimization (not just testing for testing’s sake).
7 best practices for A/B testing
When starting out with experimentation, it’s important to situate tests (and test ideas) within a larger, big picture framework. That is, which areas of your marketing funnel or product experience should take top priority? What’s the intended impact?
Knowing the answers to these questions is crucial for a multitude of reasons. For one, you’re going to have to get internal buy-in for your testing program and show how it’s moving the needle for your business. Without a clear understanding of the problem you’re trying to solve, you’ll be hobbling together a testing plan at random – which is a waste of both time and resources.
Now, let’s go a little deeper into creating an A/B testing framework.
1. Determine the goals
First things first: what's the goal of the A/B test?
When starting out, consider the overarching goals of your business. Is there enough brand awareness among your prospective customers? Is your website traffic too low? Are you trying to incorporate more cost-effective growth strategies?
Often, “trouble spots” are a great place to start A/B testing. For example, if a marketing team isn’t meeting their MQL goals, they can A/B test their landing pages by swapping out different pieces of gated content, to see which topics resonate most with their audience.
2. Understand what you want to test
When whittling down what you should test, keep your ultimate goal in mind.
Let’s say you want to A/B test an email campaign. If the goal is to improve open rates, it makes sense to experiment with the subject line. But if your team is more focused on driving event registrations, it's likely better to test out different CTAs in the body of the email.
Here are some examples what you can test across your website, email campaigns, social media posts, or design:
Headlines
CTA text
CTA button placement
Email subject lines
Images
Product descriptions
Social media copy
Google ad copy
Free trial lengths
3. Define what KPIs you’ll use
To make heads or tails of your A/B testing campaign, you’ll want to define what success looks like, which means understanding your baseline performance. What are you current metrics when it comes to paid ad campaigns, MQLs, website traffic, and so on?
Knowing where you're starting from is essential to understand how you've improved. It's also worthwhile to compare your current performance with industry benchmarks. These industry-wide averages can serve as a measuring stick of what's working and what isn't (especially compared to some of your competitors). Take website popups: according to Campaign Monitor, the average conversion rate is around 3%, with top performers reaching 10%.
A few common examples of KPIs used to measure success include:
Conversion rates
Website traffic
Revenue
Customer lifetime value
Engagement (e.g. clicks, open rates, purchase recency, etc.)
Product adoption
NPS
4. Target the right audience
There will be times when an A/B test seeks to understand the behaviors of a specific audience segment. Perhaps you want to target a specific persona that would be interested in a new product feature, or you want to exclude current customers from a paid ad campaign.
This is where audience segmentation comes into play. You can segment audiences based on behavioral data, demographics (like job title, industry, location), or by visitor source (e.g. paid ad, organic search, and so on.). We're big proponents of always considering users' behavioral and historical data when creating an audience. These two components show how people interact with your brand specifically, and provide important context.
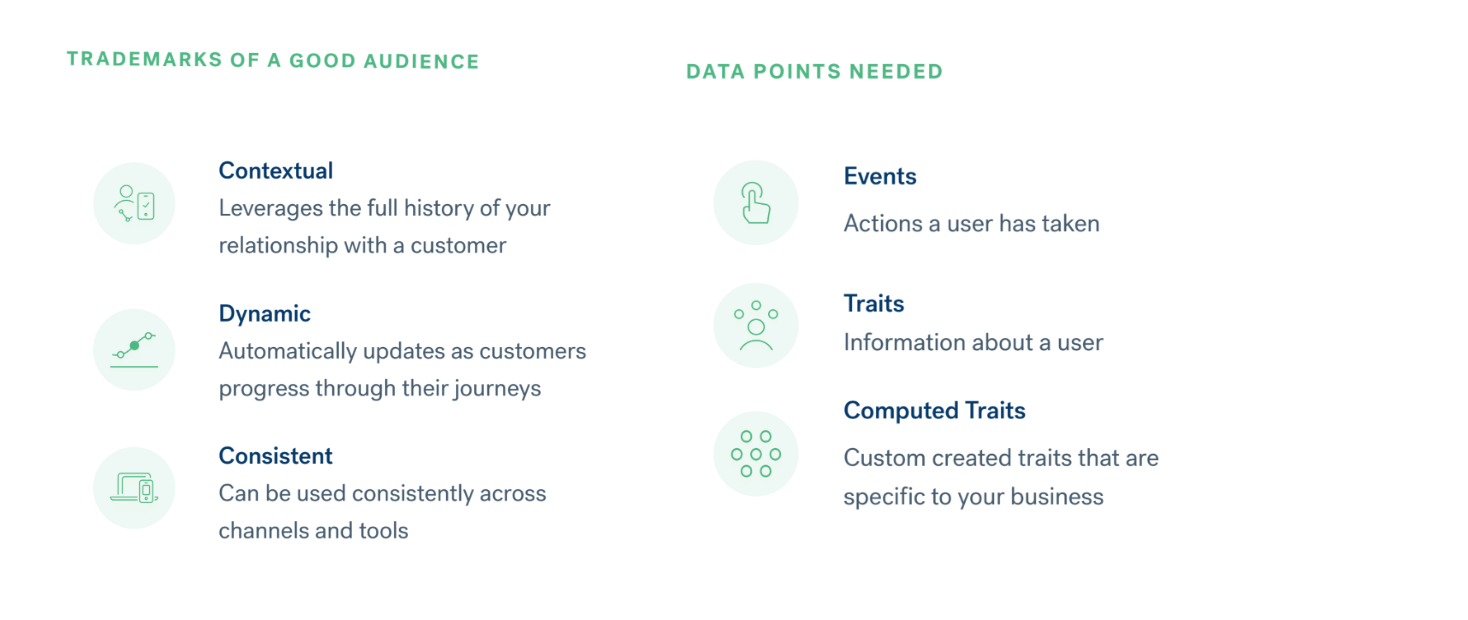
Learn more about customer segmentation here.
5. Test at the right time
The timing of your A/B test can significantly impact the results.
Figuring out the right time to test can feel like the Goldilocks’ conundrum. You don’t want to test in a slower period for your business, as you need a large enough sample size to ensure accuracy. But on the other hand, running a test during an exceptionally busy time (e.g. in the lead up to a holiday) may not reflect user behavior on the day-to-day.
To determine the right time to test, think back to the goals of the experiment and your baseline performance. If the goal is to optimize a Black Friday campaign, then testing around the holidays is the logical choice. But if the goal is to see how a UX change will impact user behavior on the day-to-day, it's best to pick a time that isn’t influenced by seasonality.
6. Ensure statistical significance
An important part of A/B testing is statistical significance, or the confirmation that the differences between group A and group B aren’t a fluke. As a quick note, a 95% confidence level is the usual benchmark for ensuring test results are accurate, which would be a p-value of 5%. (You can go deeper into the difference between these values here.)
There are free calculators available that can help you determine the sample size needed to reach statistical significance. These calculators often take into account your website traffic, conversion rate, and sometimes the Minimum Detectable Effect (MDE), which is the smallest lift/improvement that you want to detect from the experiment.
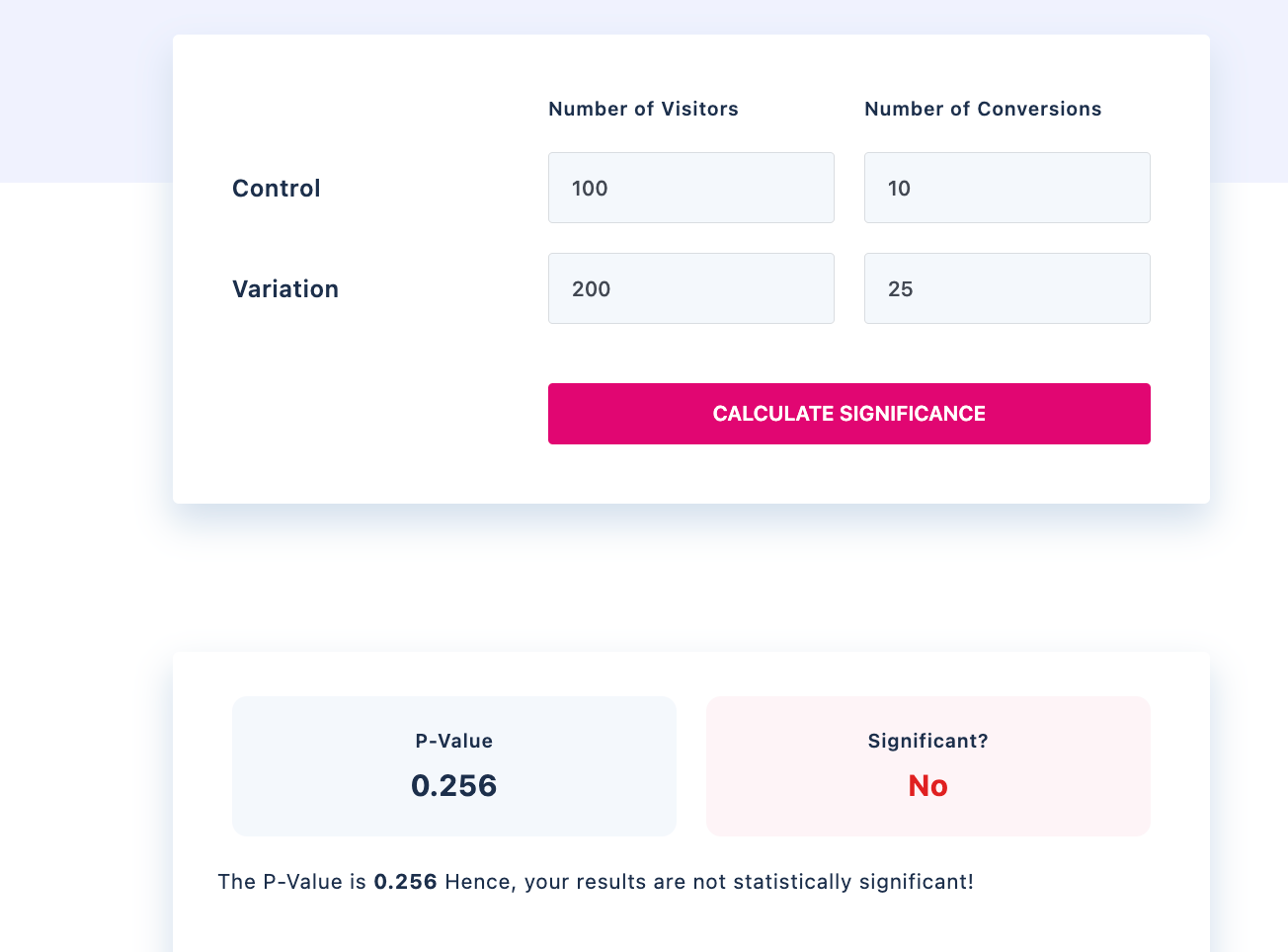
VWO’s plug-and-go significance calculator for A/B tests
7. Understand the data collected
You’ve run the A/B test, you’ve collected the data – now, you need to analyze the results. Experimentation is a pathway to understanding; even a failed experiment can provide important customer insights.
But understanding A/B test results can be complex. You have to navigate statistical significance, false positives, and ensure there hasn’t been a glitch in data collection. Luckily, there are tools that can help you set up A/B testing campaigns and safeguard against misinterpreting results.
Here’s a list of the experimentation tools that integrate with Twilio Segment.
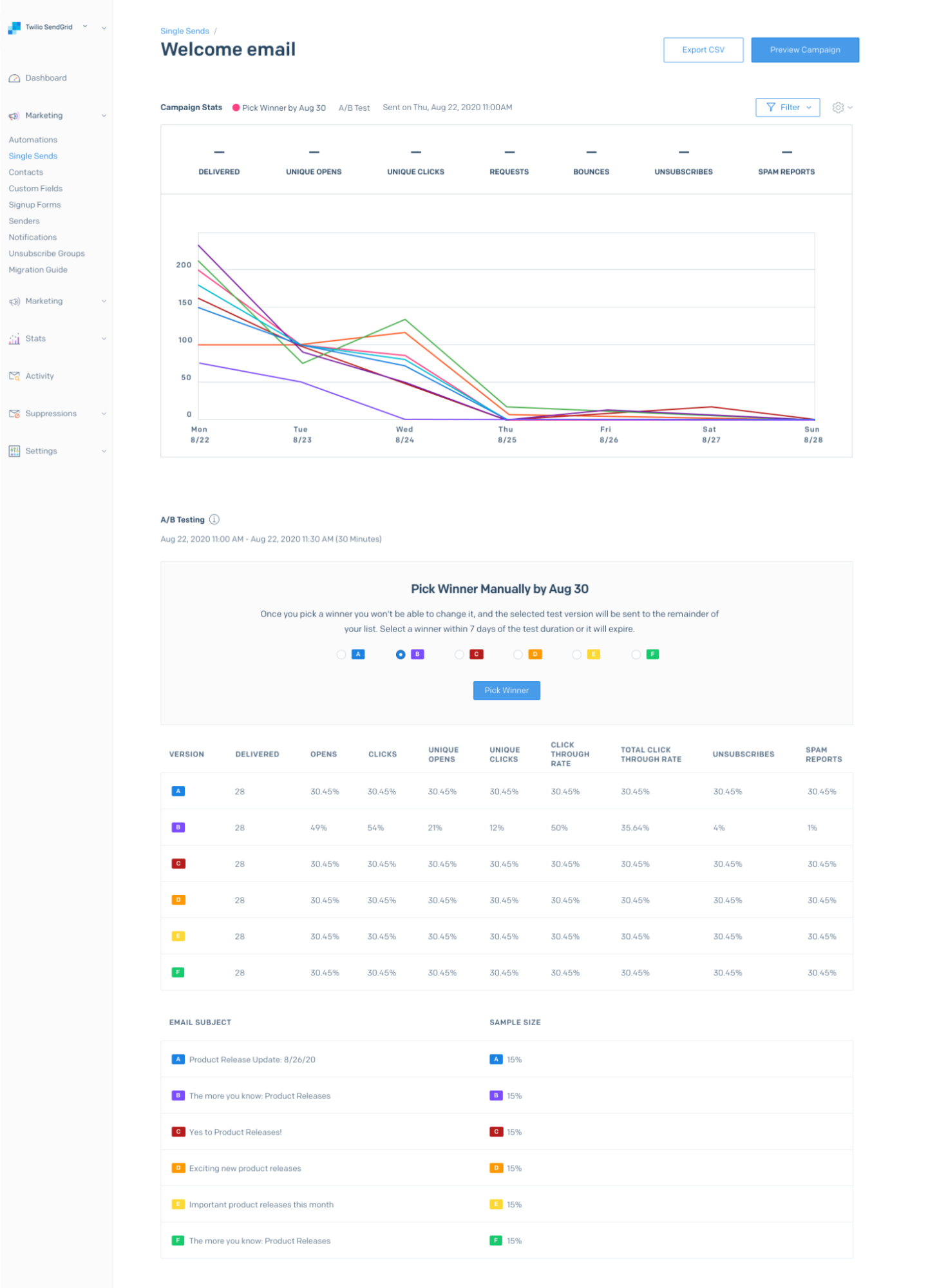
In Twilio SendGrid, you can set up A/B tests for emails to optimize open rates and engagement.
Interested in hearing more about how Segment can help you?
Connect with a Segment expert who can share more about what Segment can do for you.