What is Big Data? Definition + Guide to Big Data Systems
Dive into the world of big data. Uncover insights, enhance decision-making, and drive growth. Learn how big data can transform your business approach.
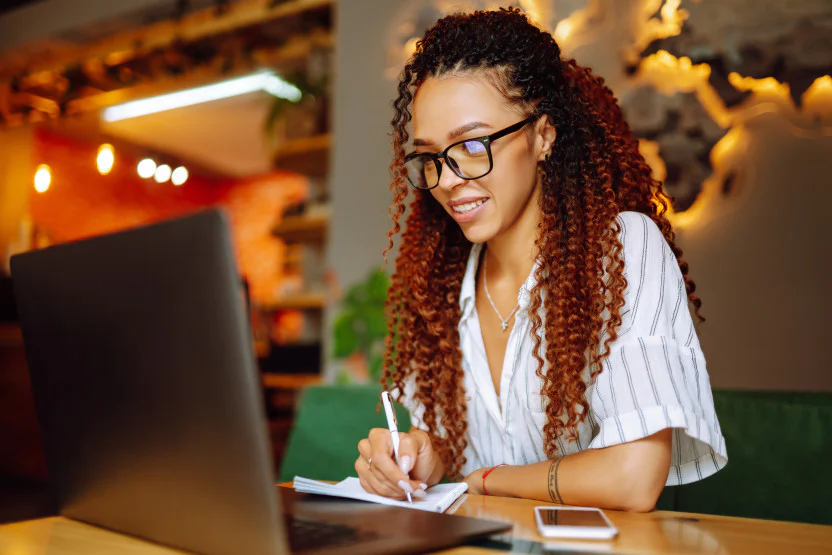
Dive into the world of big data. Uncover insights, enhance decision-making, and drive growth. Learn how big data can transform your business approach.
Big data is often characterized by “the three Vs” – volume, velocity, and variety (more on that below). True to its name, big data refers to huge, complex data sets that are not easily managed with traditional processing tools and techniques.
Structured, semi-structured, and unstructured data all fall under the umbrella of big data, and this data is often (at least in part) generated in real time (e.g., streaming data from an IoT device, like a smartwatch that tracks exercise activity and sleep patterns). As such, the volume of big data is often measured in terabytes and petabytes to exabytes. (As a frame of reference, the average company manages 162.9TB of data, a number that soars to 347.6 TB for enterprise businesses.)
A single platform to collect, unify, and connect your customer data
Roughly ten years ago, a McKinsey report predicted that big data “will become a key basis of competition, underpinning new waves of productivity growth, innovation, and consumer surplus.” Today, billions of smartphones and IoT devices are generating more data than was once thought possible, which has not only proved that McKinsey was correct, but has ushered in a unique set of challenges and opportunities that all businesses now face.
Above, we mentioned the three Vs that often define big data: volume, velocity, and variety. But data scientists have actually expanded on this list to include three more characteristics, which are: veracity, value, and variability. Let’s go through these, one by one.
Volume: Big data sets contain large quantities of data ingested from numerous sources, such as IoT devices, web browsing activity, social media, and other apps and equipment.
Velocity: Big data sources generate data in real time or near real time, requiring organizations to implement a data architecture that handles a constant stream of data.
Variety: Big data is usually a mix of structured, semi-structured, and unstructured data.
Veracity: The data may be of varying quality and require processing and integration with other data to provide value.
Value: Organizations can use insights from big data to improve products, build campaigns, and leverage them in many other ways that bring value to the business.
Variability: The stream of data is unpredictable. Specific events, such as holidays, may result in an increased data flow.
The origins of the term “big data” can be traced back to the 1990s. John Mashey, then chief scientist at Silicon Graphics, was the first to use the phrase to describe large data sets. The concept further gained prominence in the early 2000s when digital innovations became widespread, creating more data.
This led to a demand for solutions that could manage big data. In 2005, Doug Cutting and Mike Cafarella developed Hadoop, an open-source, big data storage and analysis framework. With Hadoop and big database solutions like NoSQL (“not only SQL”), organizations were able to store and analyze big data sets.
Since then, companies such as Microsoft and Amazon have launched platforms that allow companies to easily implement a big data system that stores different types of data without having to build one in-house.
The World Economic Forum estimates that by 2025, the global population will generate 463 exabytes of data daily. Organizations that are able to turn this vast amount of data into insights will drive better decision-making and performance in security, customer experience, and other business initiatives.
Cybersecurity threats are constantly evolving, demanding more sophisticated defense strategies. Big data enables cybersecurity teams to run in-depth analyses to discover anomalies that could signal an attack or breach. With these insights, they can prevent threats in real time. In banking, for example, big data can power behavioral biometrics that detect suspicious behavior before a fraudster takes advantage of a customer’s funds.
Big data powers machine learning tools that uncover new patterns or insights that enable other types of solutions. Chatbots are a widespread example of machine learning technology powered by big data – they can learn from customer conversations and improve over time. Recommendation engines, responsible for serving hyper-personalized suggestions on platforms such as Spotify and Netflix, are another result of machine learning technology.
Any business that wants to deliver innovative products needs data. With big data, businesses have the ability to tap into highly nuanced insights about customers (from their preferences, to their predicted intent, and behavioral history).
This information allows companies to see, often in real time, how people interact with their brand and use specific products or features. It presents a microview of how to personalize and improve individual experiences, and a macroview of overarching patterns and trends (e.g., seasonal dips or spikes in engagement).
Personalization isn’t just a buzzword – it provides a tangible ROI. Sixty-two percent of business leaders say personalizing the customer experience has had a positive impact on retention, and without big data, these efforts wouldn’t have been possible. The ability to unify customer data from different channels, track behavioral changes, and understand the customer’s journey helps businesses tailor their communication to maximize conversions.
The many Vs that characterize big data also add to its complexity. First, the sheer volume of data requires businesses to take a strategic approach to data integration, storage, and management. Taxfix, a Berlin-based mobile tax app, navigated these challenges by investing in a scalable cloud data architecture and a data ecosystem that unified all of its customer data.
Many organizations also default to gatekeeping data access to specific roles, creating bottlenecks when different teams need to access data for reports, marketing campaigns, and other business needs. To prevent these inefficiencies, support data democratization with self-service analytics and break down data silos.
When dealing with large quantities of data, businesses often run into issues with quality, especially if the data needs to be integrated from several sources. Without trustworthy data, departments argue over whose data is more correct, while important marketing campaigns end up unsuccessful as they are based on poor-quality customer insights.
ZALORA, an online clothing retailer, tackled unreliable customer data by first unifying data from different sources and then using it to create dynamic customer profiles. With these profiles, ZALORA could provide a high-quality shopping experience, and as a result, it doubled its conversion rates.
Lastly, the protection of customers’ privacy and regulatory compliance become more challenging as you handle more data. A big data solution that can manage personal data, automatically block the collection of certain data, and easily honor the requests of data subjects will help you avoid hot regulatory waters.
Big data is a hefty topic. To help you advance your knowledge and bring data order to your organization, refer to our guides that cover essential concepts such as data governance and data integration.
Segment’s Analytics Academy is an additional free resource that can help you broaden your knowledge through six courses. You will learn how to collect the right data, build a growth stack, and leverage data to boost revenue.
Big data refers to large amounts of structured, unstructured, and semi-structured data that are complex in volume, velocity, and variety. Due to its complexity, it cannot be handled by traditional data processing tools.
Location-based marketing is one modern example of big data in action. When you visit a store and later see Instagram ads for their products in your feed, the ads are powered by big data analysis that connects your physical location to a business address.
The main types of big data are:
Unstructured
Semi-structured
Structured
The use of big data analytics is present across industries, including transportation (supply chain management), meteorology (weather forecasting), healthcare (remote patient monitoring), and artificial intelligence (conversational bots). It allows businesses to make data-driven decisions, optimize their marketing spend, perform advanced analytics, and much more.
In simple terms, big data is large and complex data sets. Data analysts use it to discover trends in customer behavior, improve security and operational efficiency, build cost-effective marketing campaigns, and more.
In the future, big data technologies will process even greater volumes of data, driving further demand for storage systems that can handle its complexity. With more data, machine learning will be able to detect trends and glean insights with greater accuracy, creating algorithms that deliver a higher level of personalization in digital products and experiences. Organizations will also need to invest in effective data governance to effectively protect and manage their data assets.