Good Data, Better Marketing Podcast
Tune in to see how top leaders across industries are powering their customer engagement strategies with a strong data foundation.
Browse episodes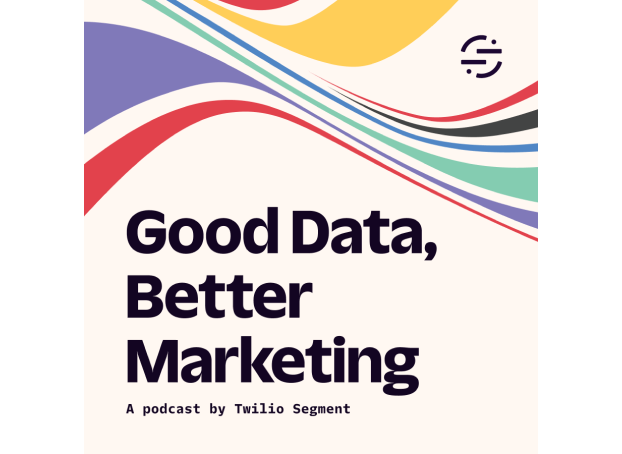
Discover what you are looking for by searching blog posts
Recent articles
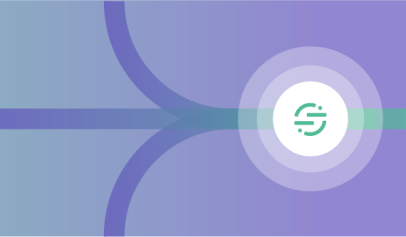
How to accelerate time-to-value with a personalized customer onboarding campaign
To help businesses reach time-to-value faster, this blog explores how tools like Twilio Segment can be used to customize onboarding to activate users immediately, optimize engagement with real-time audiences, and utilize NPS for deeper customer insights.
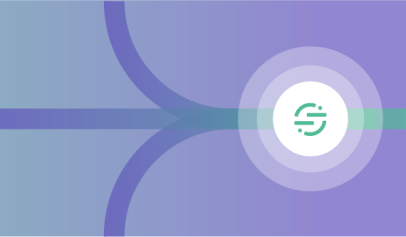
Introducing Segment Community: A central hub to connect, learn, share and innovate
Dive into Segment's vibrant customer community, where you can connect with peers, gain exclusive insights, and elevate your success with expert guidance and resources!
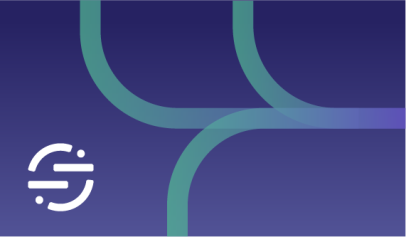
Using ClickHouse to count unique users at scale
By implementing semantic sharding and optimizing filtering and grouping with ClickHouse, we transformed query times from minutes to seconds, ensuring efficient handling of high-volume journeys in production while paving the way for future enhancements.
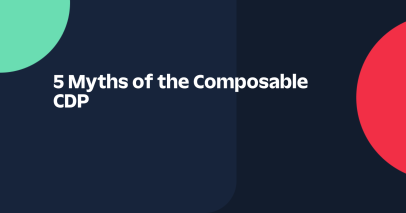
5 Myths of the Composable CDP
Composable CDP - fact or fiction? We break down the five most popular myths.
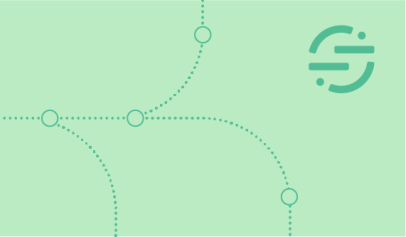
Accelerating your time to value with effective Observability
Twilio Segment provides a comprehensive suite of tools for efficiently managing, activating, and analyzing your organization's data, ensuring transparency and empowering you to unlock its full potential effortlessly.
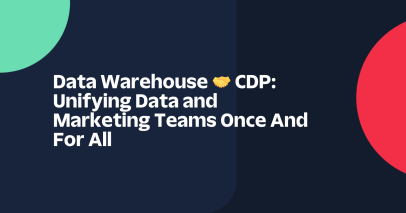
Data Warehouse + CDP: Unifying Data and Marketing Teams Once And For All
We explore how a customer data platform (CDP), alongside your data warehouse, keeps marketing and data teams on the same page.
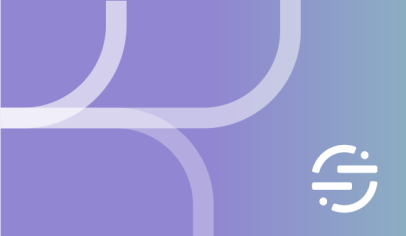
Unlock next-level insights: Introducing Segment Reverse ETL with Snowflake Cortex
Discover how Segment's Reverse ETL integration with Snowflake Cortex improves data management, enabling effortless integration and transformation for enhanced analytics and operational efficiency.
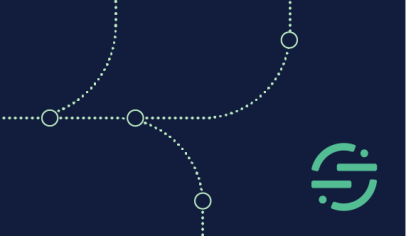
Terraform and CDP: Manage your data infra as code
Segment Terraform is now Generally Available.
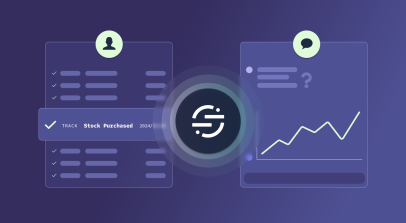
What did you say to me? Instrumenting User Insights for your AI Copilot
Cultivating a symbiotic relationship between user insights and AI copilots, we delve into the art of instrumenting seamless collaboration for enhanced performance and user satisfaction.
| Articles by topic
Engineers & Developers
Showcasing solutions and best practices of Segment engineers using some of our favorite tools.
Using ClickHouse to count unique users at scale
Accelerating your time to value with effective Observability
Growth & Marketing
Give your customers the personalized marketing they want, and grow your business to scale.
How to accelerate time-to-value with a personalized customer onboarding campaign
Individualization without cookies: The missing ingredient
Customer Data & Analytics
Understand the needs of your customers by analyzing how they interact with your website, app, and product.
Reengaging churned customers: 3 ways to win them back
4 things we learned at CDP Live 2024
Unlocking the Power of Facebook's Conversions API with Segment: A Guide to First-Party Data Retargeting
From Cookies to Consent: Addressing Advertiser Challenges in the Era of Privacy Changes
Customer Data Platform
Best practices, tips, and tricks to help you harness the potential of your customer data platform.
5 Myths of the Composable CDP
Data Warehouse + CDP: Unifying Data and Marketing Teams Once And For All
Introducing Segment Community: A central hub to connect, learn, share and innovate
Twilio Segment expands omnichannel AdTech capabilities with generative AI, first-party profile data enrichment, and Consent Enforcement to maximize advertising reach and impact
Identity Management
Learn how to build a complete view of the customer from data across multiple touchpoints.
Leveling Up Identity Resolution: The Four Biggest Pitfalls of DIY Identity Resolution
Leveling Up Identity Resolution: How Identity Resolution Lays the Foundation for Complex Customer Engagement Solutions
Personalization
Best practices for using customer data to power customized, personalized user experiences.