Engineers & Developers
A blog for engineers, by engineers
Showcasing solutions and best practices of Segment engineers using some of our favorite tools.
Engineers & Developers
Tracking Meaningful Security Product Metrics
Meaningful security metrics demonstrate that your security organization is mitigating risk and driving sales.
Leif Dreizler • Dec 8, 2022
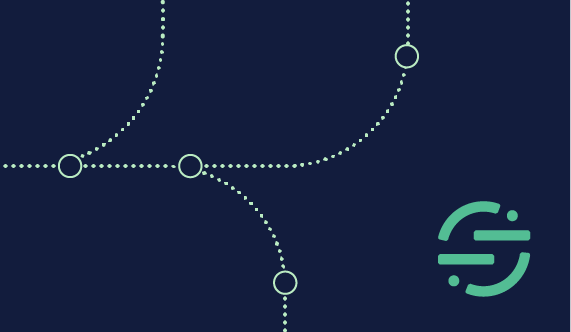