You don’t need to look very hard to find research supporting the argument that bad data is detrimental to companies and organizations. Comprehensive studies tell us that bad data is no longer simply a matter of improving operational efficiency but also a mission critical requirement.
If the median Fortune 1000 business increased the usability of its data by just 10%, it would translate to an increase in $2.01 billion in total revenue every year. — University of Texas
Bad data costs the U.S. $3 trillion per year. — Harvard Business Review
The executives surveyed by PwC said cleaning up their data will lead to average cost savings of 33%, while boosting revenue by an average of 31%. — Wall Street Journal
I’m part of the Solutions Architect team at Segment. Our team ensures that our enterprise customers successfully implement our platform. Through my experience over the last several years, I’ve seen firsthand just how severe the impact of bad data can be, and it’s not specific to any one industry or organization size.
My team gets an intimate view into how bad data hinders many companies’ abilities to communicate with their customers, power reliable analytics, and drive measurable business impact. When left unaddressed, bad data has both short and long-term effects on a company’s bottom line.
In this article, I’ll share some observations about what bad data looks like and give you tips on how the best companies prevent bad data in the first place.
What is bad data?
Bad data doesn’t always start off bad. Many times it was good data that had something bad happen to it. (The poor data!)
If we consider bad data to be an outcome, or a byproduct, then what are the causes of it? Here are the markers of what we’ve come to identify as “bad” data.
Stale data is bad data
Stale data sounds like: “This is last month’s data. Where’s today’s report?”
More and more critical business use-cases powered by customer data require the data to be readily available in almost real-time. This is a need across most modern organizational functions—from Marketing, to Sales, to Analytics, to Product, to Finance.
Teams need fresh customer data as quickly as possible so they can make informed decisions or deliver personalized experiences to their customers. Here are a few scenarios when data needs to be ready fast.
-
Personalization: In this context, “personalization” refers to the application of data to maintain a highly relevant relationship between a company and its customers. This has become an entry-level requirement for businesses in many industries, especially in eCommerce and Media, to remain competitive. Personalizing a customer’s experience—from initial contact on the web or mobile, all the way to customer re-engagement through email or push notification—requires data points be updated and refreshed as often as possible.
-
Analytics: Fresh, timely data has also become necessary for informing good decision-making at organizations across industry sectors. For example, real-time analytics informs supply-chain logistics and both short and long-term product development. It’s used to drive real-time product merchandising, content planning, and much more.
Inaccessible data is bad data
Inaccessible data sounds like: “I have to wait three months to launch the campaign because I can’t get my hands on the right data.”
Another key indicator of bad data is when it’s inaccessible to the teams within a company that need it the most. I’ve found that the inability to access the right data becomes an increasingly important issue the larger and more distributed an organization becomes. This is often referred to as the “data silos” problem, where information is not available across business units or teams.
Data silos can be created by system permission issues, incompatible schemas, sprawling tool stacks, or different data sources populating different environments. To unify the silos, many companies have embarked on data lake projects over the past few years that pull together all data across a company into one warehouse. However, this approach doesn’t address the accessibility issue because only specialized technical teams can extract data from a data lake. A common infrastructure powering each department’s tools—and the data lake—with the same data can be a good solution.
Confusing data is bad data
Confusing data sounds like: “Is there anyone in our department who knows what the data points in our tables actually mean? I just want to run a quick update to this report.”
In order for data to be useful, it needs to be clearly understood. I’ve partnered with companies where existing data capture methods were set up haphazardly without a clear system anyone could use to understand what the data actually means. This approach results in only a limited number of people knowing how to interpret the data.
Having a clear, declarative format for naming and describing your data helps reduce the additional process of “cleaning” or “scrubbing” it before internal teams can use it. A simplified, easy-to-understand tagging framework for naming customer data is essential for democratizing data-driven decisions. It’s also important to make a central repository that houses this information accessible to anyone that needs to use the data.
Disrespectful data is bad data
Disrespectful data sounds like: “An angry prospective customer is asking why we sent this message when they never asked to receive information from us.”
Consumer privacy has become critical for companies of all sizes. A decade ago, abundant consumer data collection by internet services and applications was viewed as an asset. Today the sentiment has shifted, and customer data collection without the right controls has turned into a liability. As a result, bad data looks like data that’s not collected with consent and is not used in accordance with a customer’s expressed preferences.
To comply with regulations like the GDPR and CCPA, not only do you need to collect and use data with consent, you also need an easy way to delete or suppress a customer’s information if they ask. This is hard to wrangle without a consolidated data infrastructure.
Using third-party data that’s purchased via data brokers and intermingled across companies exacerbates this problem because it’s hard to accurately collect consent for third-party data. Optimizing experiences with first-party data, or data only used between a customer and the company they interact with, is a more respectful approach.
Untrustworthy data is bad data
Untrustworthy data sounds like: “Are you sure these numbers are correct? They don’t match up with my analysis.”
There are many instances in an organization that can cause data distrust. A report might feel off, and after some digging, you find the data source has been corrupted or stopped collecting altogether. Different tools and dashboards might read different results for the same question, prompting more questions, and grinding business to a halt. Any data discrepancy can cause a huge business impact, not just in the time spent to track it down, but also potential revenue lost by triggering poor customer experiences or making the wrong business decision.
The best approach, described by DalleMule and Davenport in their Organizational Data Strategy HBR article, is to have one source of truth for the business that allows each team and department to create a view of the data that works for them.
Turning around your bad data
Now that we know what bad data is and its consequences, how exactly do companies begin to improve their data practices?
First, it’s important to acknowledge that having bad data is actually the default state of a company. Without proactive processes and infrastructure in place, your data will degrade into chaos.
Consider the sheer amount of data generated today—over 90% of the data in existence today was created less than 2 years ago. While an abundance of customer data might give an organization a potential leg-up for things like machine learning and individual personalization, it’s also become a Herculean task to organize it, clean it, process it, and catalog it. This is why, here at Segment, we advocate for our customers and partners to take a deliberate and opinionated approach to their customer data.
Here are some of the best data strategy and management patterns I’ve observed in partnering with some of the world’s most forward-thinking enterprise companies.
Treat data like a product
When companies truly operationalize data, they treat their data infrastructure like a product that’s properly staffed, monitored, and maintained like a production-grade system. They appoint a single responsible executive-level individual such as a CIO or CDIO (Chief Digital Information Officer), and that person has a dedicated cross-functional team of product managers, engineers, and analysts.
These organizations implement a unified, central data governance strategy across business units and product teams.
Balance standardization and flexibility
Strong organizations seek to achieve a data strategy that balances standardization and flexibility. Standardization is key, so that all teams can coordinate using a shared understanding of that data’s truth (i.e., we trust the data we have to work with). Flexibility, on the other hand, is necessary to accommodate individual teams using the data to suit their needs with the tools they prefer to use.
If you are completely rigid in how your teams use data without accounting for their needs, departments will go rogue and create siloed data as previously discussed. However, if you don’t give them any parameters for how to use data respectfully and with a common framework, you’ll never be able to do higher level analysis across products, platforms, and business units.
Routinely audit your data stack
New solutions to address data problems crop up almost weekly (see this analysis on the martech landscape), and the best companies can easily test and try new tools rather than focusing on cleaning up their existing mess. Organizations that I consider industry leaders implement a data infrastructure that enables them to adopt new technologies and have long-term flexibility as requests for new tools and systems get introduced into the market.
These organizations are also adopting practices and tooling to automate auditing data. This includes flagging and blocking bad data at the source and enforcing a predefined data specification so they can trust the data in each tool they use.
Build a culture around documentation
Strong knowledge management, sharing, and accessibility is more critical than ever. At the end of the day, a company is simply an aggregation of people working together toward common goals. The larger the company, the more information is generated, and the harder it is to communicate what’s important and what’s not while the organizational stakes often get higher.
Clear channels for sharing what your data practice looks like, guidance for how to capture data, and rules on how to effectively and securely share data with those who need it, are all critical components that lead to success.
It’s time to say goodbye to bad data
Here at Segment we’re always looking to deliver useful products and tooling to help customers observe, evaluate, and act to fix their bad data. Our platform helps organizations of every size take a proactive approach to good data by helping them monitor the data they capture, enforce standard data practices, and offer every team and tool access to the same, consistent first-party data.
We believe good data is Segment data. In our next blog post we’ll dive even deeper into how you can achieve good data using Segment.
Want to learn how to turn your bad data around so you can make business decisions you trust? Reach out. We’re happy to discuss how we can help!
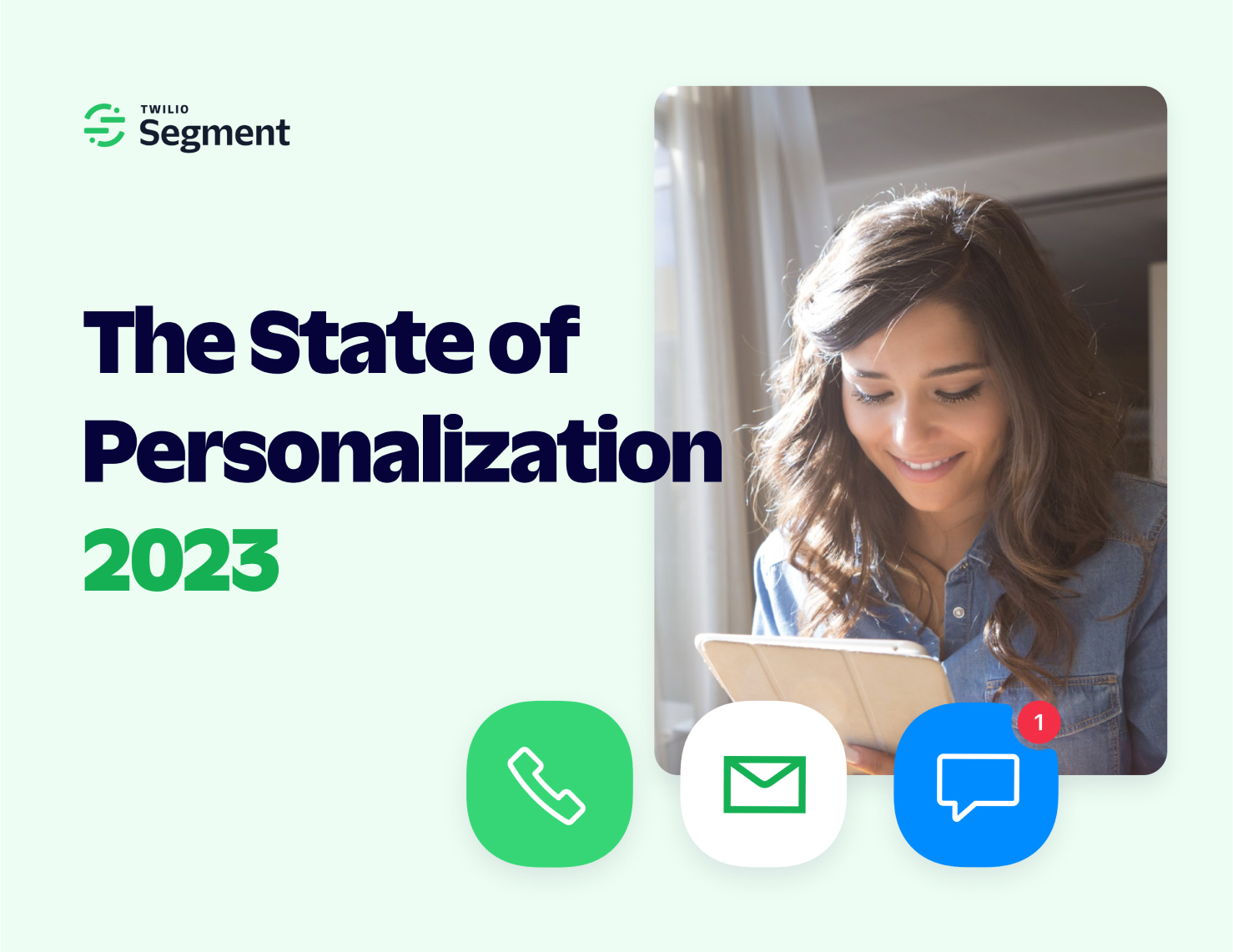
The State of Personalization 2023
Our annual look at how attitudes, preferences, and experiences with personalization have evolved over the past year.